Share this
Fiona Macfarlane
University of St. Andrews, Scotland, frm3@st-andrews.ac.uk"Bridging the gap between individual-based and continuum models of growing cell populations"
Alexey Penenko
ICM&MG SB RAS, Russian Federation, a.penenko@yandex.ru"Inverse Modeling of Biological Processes with Adjoint Ensemble Methods"
Karina Islas Rios
Monash University Australia karina.islasrios@monash.edu"NetScan: a computational tool for discovering and visualizing biochemical networks with defined topological structures."
Hyukpyo Hong
Korea Advanced Institute of Science and Technology, Republic of Korea, hphong@kaist.ac.kr"Derivation of stationary distributions of biochemical reaction networks via structure transformation"
Linard Hoessly
University of Copenhagen, Denmark, hoessly@math.ku.dk"Stationary distributions of stochastic reaction networks via decomposition"
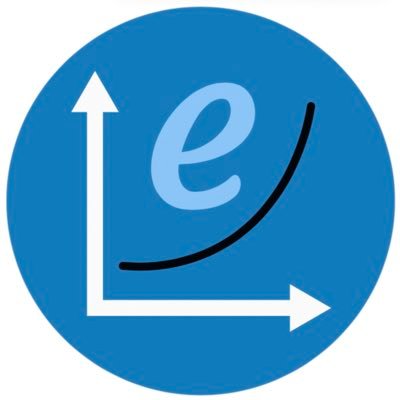