Poster
A combined systems biology and machine learning approach to study patient response to anti-PD1 immunotherapy
Share this
Michelle Przedborski
University of Waterloo"A combined systems biology and machine learning approach to study patient response to anti-PD1 immunotherapy"
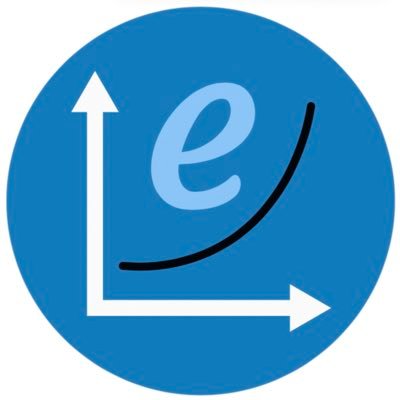