Share this
Kevin Hannay
University of Michigan"Circadian State Estimation using Wearable Data"
Maia Angelova
Deakin University"Data driven model for detecting insomnia from multi-night actigraphy time series data"
Davide Maestrini
UCLA"A mathematical model of 'wanting', 'liking, and brain reward circuitry in drug addiction"
Paul Roberts
University of Sussex"Using mathematics to investigate the mechanisms behind vision loss"
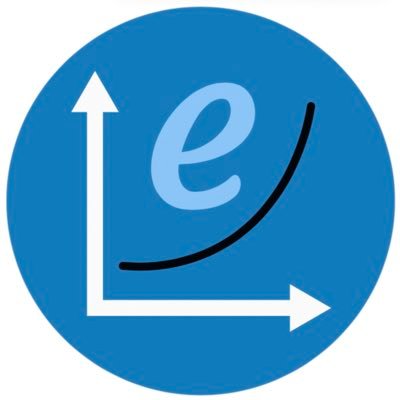