Organizers:
Heiko Enderling, Alexander R.A. Anderson
Description:
The mathematical oncology subgroup of the Society for Mathematical Biology is growing, reflecting the increasing number of scientific publications on the mathematical modeling of cancer. Cancer models are being developed to answer a diversity of questions, that cover almost every cancer type and stage, including how we might better understand or treat this deadly disease. The specific modelling approach used varies widely, while some models are phenomenological in nature following a bottom-up approach, other models are more top-down data-driven. This two-part minisymposium showcases the breadth of mathematical oncology research from scientists at both Universities and Hospitals at different career stages form around the world to spur discussions and collaborations in the field of mathematical modeling of cancer.
Mohit Kumar Jolly
Indian Institute of Science, India"Integrating mechanism-based and data-based approaches to identify hybrid epithelial/mesenchymal phenotypes"
Pamela Jackson
Mayo Clinic, Phoenix, USA"Parameterizing a Brain Tumor Growth Model Using Noisy Simulated MRIs"
Jeffrey West
Moffitt Cancer Center, Tampa, USA"Anti-fragile Cancer Therapy"
Anna Marciniak-Czochra
University of Heidelberg, Germany"Stem cell niche dynamics in acute leukemia: Insights from mathematical modeling"
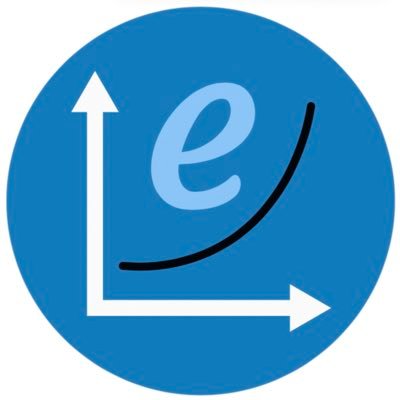