Organizers:
John Nardini and Maria-Veronica Ciocanel
Description:
The era of big data has challenged researchers to develop novel methods to succinctly summarize and analyze complex datasets. Such methods include network and topological data analysis (TDA), which use methods from graph theory, dynamical systems, and topology to describe the patterns underlying a given data set over different scales. These areas of data science have proven successful for analyzing many biological phenomena, including disease transmission, ecological swarming, medical diagnostics, and within-cell protein interactions. In this minisymposium, the speakers will 1. introduce scholars to the basic principles behind persistent homology (a popular and useful technique of TDA) and 2. highlight current challenges and key areas of using TDA to inform mathematical modeling. In particular, their talks will highlight recent studies that have shown that this area of data science can provide informative summaries for mathematical models mimicking biological phenomena, including pattern formation and random rod networks.
Samuel Heroy
University of Oxford, United Kingdom, samuel.heroy@maths.ox.ac.uk"Rigidity percolation in random rod networks"
Yu-Min Chung
UNC Greensboro, United States, y_chung2@uncg.edu"On the morphology of mitochondria via a multi-parameter persistent homology approach"
Alexandria Volkenning
Northwestern University, United States, alexandria.volkening@northwestern.edu"Topological data analysis of zebrafish skin patterns"
Ashish Raj
UCSF, United States, ashish.raj@ucsf.edu"Inference on models of network spread and protein aggregation in Alzheimer’s and dementia"
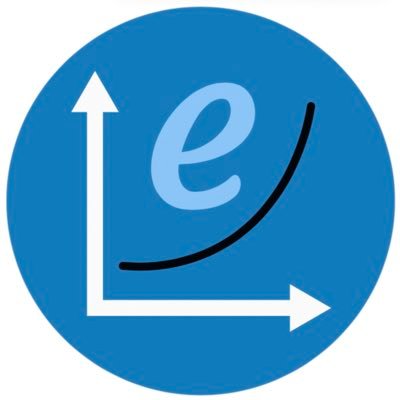