Share this
Anne Talkington
Chapel Hill"A PBPK model for clearance of PEGylated nanomedicines"
Tatiana Marquez-Lago
UAB"Mathematical methods for microbiome research"
Camile Kunz
Goethe U."Chemotaxis impact on pattern formationChemotaxis impact on pattern formation"
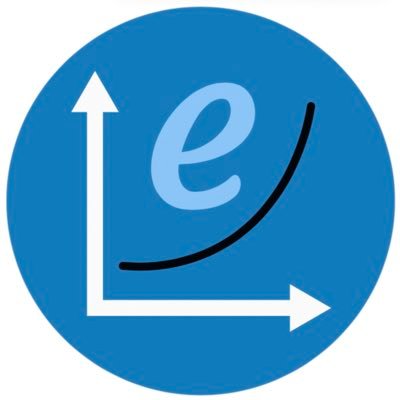